- September 29, 2022
- Posted by: Admin
- Category: Artificial Intelligence, Technology
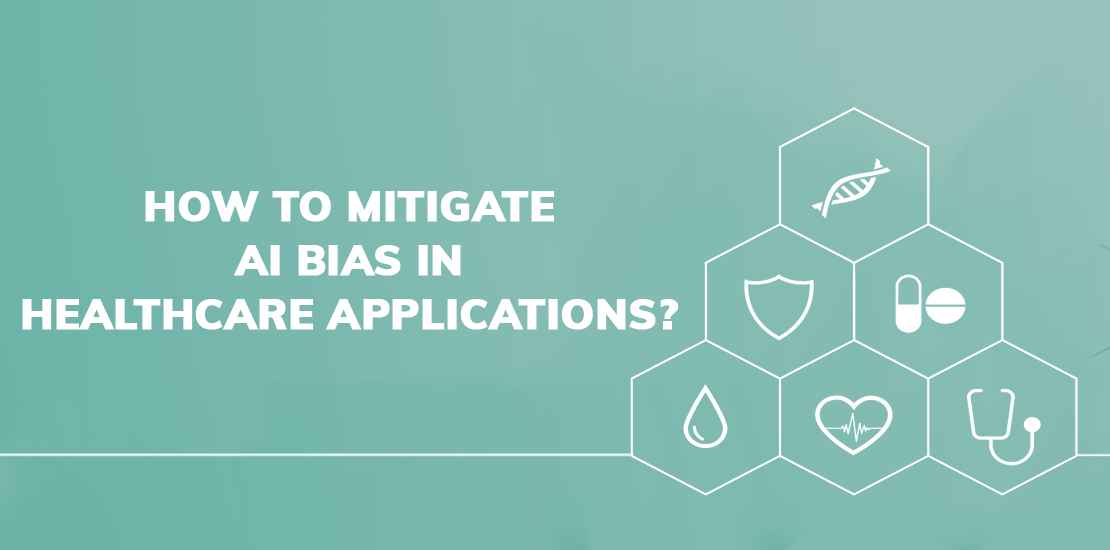
Artificial intelligence keeps inching its way into more and more aspects of our life, greatly benefitting the world. AI technology advancements are finding intriguing applications in healthcare as well. Medical institutions have begun to improve their infrastructure by reacting to technological developments.
Healthcare organizations must make a concerted effort to mitigate AI bias in healthcare applications because a biased AI model can seriously harm patients’ health and well-being. But, how can we prevent algorithmic bias in healthcare?
Keep reading to find the solutions for avoiding AI bias in healthcare.
What is bias, and how does it affect patient well-being?
The issue of bias in the medical industry is not something new. We can see the occurrence of bias when there is discrimination against a particular population segment – either consciously through preconceived notions like racism and sexism, unconsciously through ingrained thoughts based on assumptions or stereotypes, or inadvertently through the use of data skewed towards a particular segment of the population.
A biased AI solution often leads to incorrect diagnoses and patient care recommendations. The development of any solution powered by artificial intelligence begins with data. However, healthcare data has an abundance of privacy and security issues associated with it. A recent study found that institutes that share medical data are more likely to lose patients as compared to those institutions that keep their patient data confidential. This makes it extremely difficult for solution developers to gather the necessary data to build an accurate AI model, which leads to poor quality solutions that can often cause errors during diagnosis.
How does an AI solution become biased?
Artificial Intelligence relies on data input to train machine algorithms to make decisions. Millions of data must to be fed to distinguish between the target groups and contributing factors. From this, the machine learns how to create relevant distinctions. The AI algorithms cannot produce accurate outputs if the underlying data is inherently biased or doesn’t contain a diverse representation of target groups.
AI solutions created by humans will always be biased based on their understanding. Machine learning models can reflect the biases of organizational teams, designers in the group, the data scientists who implement the models, and the data engineers who gather the data. The algorithms are inadvertently trained with data that is subjected to unintentional biases. So it will have an adverse effect on the underrepresented groups. Such unintentional biases can be observed in the various AI development and deployment phases. The source of these biases could be the use of biased datasets or the application of an algorithm in a different context than originally intended.
Ways to mitigate bias in healthcare applications
To mitigate AI bias in healthcare applications, and keep it from worsening existing inequalities, it is essential to understand the bias that seeps into an algorithm.
A few key points to keep in mind while resolving Artificial Intelligence bias in healthcare are:
Analyze your algorithm while considering the possibilities of bias
The first step in mitigating bias in a machine is to anticipate the possibility of its occurrence in an algorithm. Examine the proposed algorithm for potential sources of bias that might lead to disparate results when tested on a target population.
Search for any existing flaws in your data set
The information you get will not always be completely correct. Thoroughly analyze each piece of information and look for imperfections or mistakes that could lead to incorrect output. If you are building an AI algorithm to detect signs of mental disorders, you need to include extensive information on people suffering from depression. However, the number of data on patients diagnosed with schizophrenia may be much lower. This could be considered a significant flaw in your data set.
Take a conscious effort to guarantee fairness
Several factors need to be considered while looking for data sets to take steps to mitigate algorithmic bias in healthcare. Make sure you believe these factors to ensure fairness across different population segments. An effective way to do this would involve adjusting thresholds within the model to ensure equal outcomes and allocation of healthcare resources.
Continue to monitor the algorithm, even after it is deployed
To develop an effective AI model, you must remember that your work doesn’t just end after deployment. Keep monitoring the data for any discrepancies you may have missed during the development process. Testing a model in a controlled setting differs from deploying it in a real-life scenario.
Get feedback from healthcare officials and patients
To acquire the best feedback for your model, you must first understand the errors in the output and should try to rectify them.
Diversify your workforce
A homogenous workforce is one of the main reasons for the inclusion of bias during the development of a model. When hiring your development team, try to diversify your workforce and include people from various segments of the population. This promotes an inclusive culture where you are reducing the chances of bias during development.
Collaborate knowledge from technical and medical experts
Promote collaboration between your solution development team and healthcare experts. This can help you integrate a contextual understanding of medical care into your AI solutions. For instance, as mentioned earlier, many diseases manifest differently in various ethnic groups and genders. By integrating the knowledge of medical experts, it will be much easier to anticipate such cases and act accordingly.
Diversify your dataset
If your data sets are of poor quality, the output of your AI algorithm will suffer. To mitigate bias in healthcare algorithms you need to include well-annotated and curated datasets inclusive of all population segments.
Ensure diversity invalidation
The developed AI algorithm needs thorough validation to ensure that it executes tasks as required. This means it needs to be assessed using traditional accuracy metrics and relevant fairness metrics. There is a significant chance that your algorithm needs to be retrained and recalibrated when applied to patients across different countries or ethnicities.
As AI solutions are becoming increasingly prevalent in healthcare, organizations must take approaches to eliminate bias in healthcare AI. We need better procedures to weigh the dangers and advantages of AI technology if we want to produce a more equal healthcare solution. A collaboration between data scientists, healthcare providers, consumers, and regulators can fulfill this goal. To develop an ethical AI ecosystem that will improve medical care, medical institutions and organizations must begin analyzing AI solutions for bias and fairness. This can help mitigate bias and provide top-notch medical care to patients worldwide.